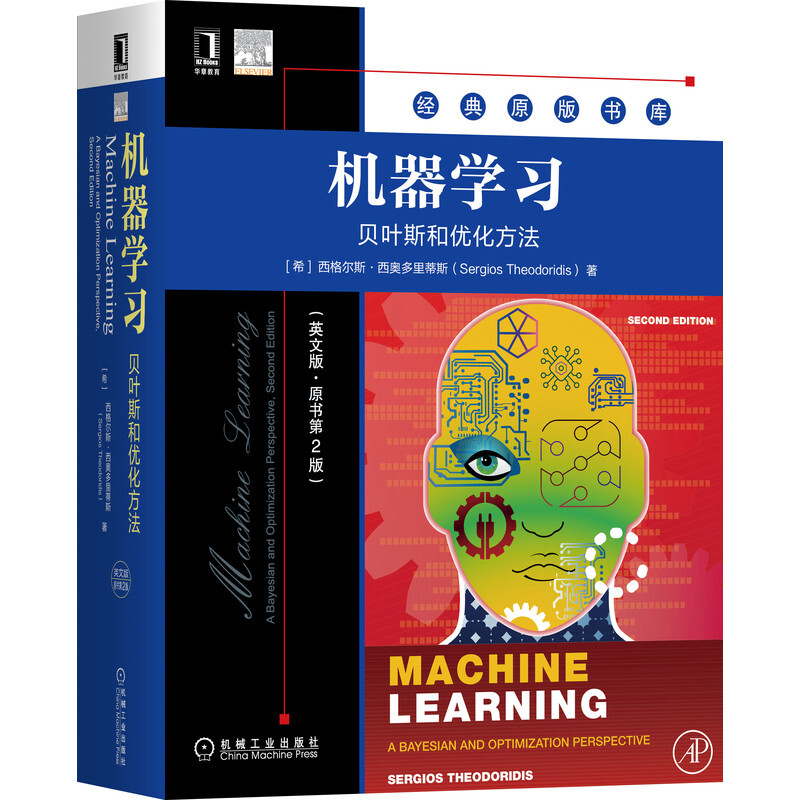
经典原版书库机器学习:贝叶斯和优化方法(英文版 原书第2版)

- ISBN:9787111668374
- 装帧:一般胶版纸
- 册数:暂无
- 重量:暂无
- 开本:16开
- 页数:1152
- 出版时间:2020-12-01
- 条形码:9787111668374 ; 978-7-111-66837-4
本书特色
全景式呈现机器学习方法和新研究趋势,重写了关于神经网络和深度学习的章节,扩展了关于贝叶斯学习的内容
内容简介
本书通过讲解监督学习的两大支柱——回归和分类——将机器学习纳入统一视角展开讨论。书中首先讨论基础知识,包括均方、*小二乘和*大似然方法、岭回归、贝叶斯决策理论分类、逻辑回归和决策树。然后介绍新近的技术,包括稀疏建模方法,再生核希尔伯特空间中的学习、支持向量机中的学习、关注EM算法的贝叶斯推理及其近似推理变分版本、蒙特卡罗方法、聚焦于贝叶斯网络的概率图模型、隐马尔科夫模型和粒子滤波。此外,本书还深入讨论了降维和隐藏变量建模。全书以关于神经网络和深度学习架构的扩展章节结束。此外,书中还讨论了统计参数估计、维纳和卡尔曼滤波、凸性和凸优化的基础知识,其中,用一章介绍了随机逼近和梯度下降族的算法,并提出了分布式优化的相关概念、算法和在线学习技术。
目录
Acknowledgmentsvi
About the Authorviii
Notationix
CHAPTER1 Introduction1
11 The Historical Context1
12 Artificia Intelligenceand Machine Learning2
13 Algorithms Can Learn WhatIs Hidden in the Data4
14 Typical Applications of Machine Learning6
Speech Recognition6
Computer Vision6
Multimodal Data6
Natural Language Processing7
Robotics7
Autonomous Cars7
Challenges for the Future8
15 Machine Learning: Major Directions8
151 Supervised Learning8
16 Unsupervised and Semisupervised Learning11
17 Structure and a Road Map of the Book12
References16
CHAPTER2 Probability and Stochastic Processes19
21 Introduction20
22 Probability and Random Variables20
221 Probability20
222 Discrete Random Variables22
223 Continuous Random Variables24
224 Meanand Variance25
225 Transformation of Random Variables28
23 Examples of Distributions29
231 Discrete Variables29
232 Continuous Variables32
24 Stochastic Processes41
241 First-and Second-Order Statistics42
242 Stationarity and Ergodicity43
243 Power Spectral Density46
244 Autoregressive Models51
25 Information Theory54
251 Discrete Random Variables56
252 Continuous Random Variables59
26 Stochastic Convergence61
Convergence Everywhere62
Convergence Almost Everywhere62
Convergence in the Mean-Square Sense62
Convergence in Probability63
Convergence in Distribution63
Problems63
References65
CHAPTER3 Learning in Parametric Modeling: Basic Concepts and Directions67
31 Introduction67
32 Parameter Estimation: the Deterministic Point of View68
33 Linear Regression71
34Classifcation75
Generative Versus Discriminative Learning78
35 Biased Versus Unbiased Estimation80
351 Biased or Unbiased Estimation?81
36 The Cram閞朢ao Lower Bound83
37 Suffcient Statistic87
38 Regularization89
Inverse Probl
作者简介
西格尔斯·西奥多里蒂斯(Sergios Theodoridis) 雅典大学教授,香港中文大学(深圳)教授,研究兴趣包括机器学习、模式识别和信号处理等。他是IEEE Fellow、IET Fellow、EURASIP Fellow,曾任IEEE信号处理协会副主席、EURASIP主席以及IEEE Transactions on Signal Processing主编。曾获2017年EURASIP Athanasios Papoulis奖,2014年IEEE信号处理杂志*佳论文奖,以及2014年EURASIP*有价值服务奖等。此外,他还是经典著作《模式识别》的作者。
-
深度学习的数学
¥43.5¥69.0 -
全图解零基础word excel ppt 应用教程
¥19.0¥48.0 -
机器学习
¥59.4¥108.0 -
有限与无限的游戏:一个哲学家眼中的竞技世界
¥37.4¥68.0 -
智能硬件项目教程:基于ARDUINO(第2版)
¥31.9¥65.0 -
硅谷之火-人与计算机的未来
¥14.3¥39.8 -
元启发式算法与背包问题研究
¥38.2¥49.0 -
AI虚拟数字人:商业模式+形象创建+视频直播+案例应用
¥62.9¥89.8 -
UNIX环境高级编程(第3版)
¥164.9¥229.0 -
剪映AI
¥52.8¥88.0 -
深度学习高手笔记 卷2:经典应用
¥90.9¥129.8 -
纹样之美:中国传统经典纹样速查手册
¥76.3¥109.0 -
UG NX 12.0数控编程
¥22.1¥45.0 -
MATLAB计算机视觉与深度学习实战(第2版)
¥90.9¥128.0 -
界面交互设计理论研究
¥30.8¥56.0 -
微机组装与系统维护技术教程(第二版)
¥37.8¥43.0 -
明解C语言:实践篇
¥62.9¥89.8 -
Linux服务器架设实战(Linux典藏大系)
¥83.3¥119.0 -
Visual Basic 语言程序设计基础(第6版)
¥32.0¥45.0 -
贝叶斯推理与机器学习
¥139.3¥199.0