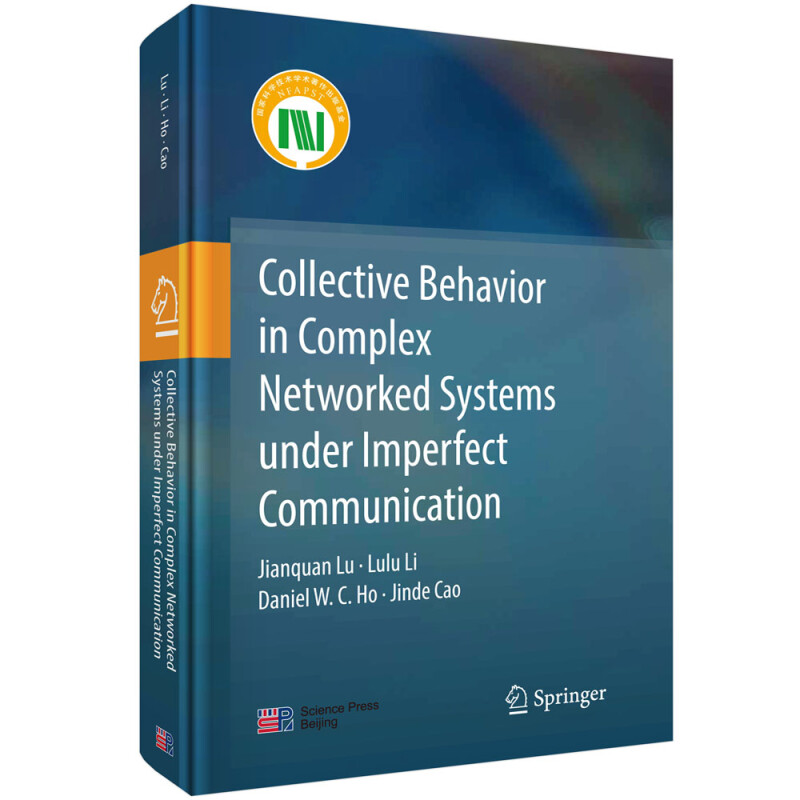
包邮通信约束下复杂网络化系统的群体行为(英文版)

- ISBN:9787030693846
- 装帧:一般胶版纸
- 册数:暂无
- 重量:暂无
- 开本:B5
- 页数:260
- 出版时间:2021-08-01
- 条形码:9787030693846 ; 978-7-03-069384-6
本书特色
书中内容被诸多学者引用和关注,推动网络群体行为和网络群体智能的发展和融合
内容简介
对网络群体行为的研究已经成为已成为21世纪网络科学研究的热门问题。在网络化系统中,由于节点间的信息传递,系统整体会呈现出群体行为,比如同步或一致性。需要指出的是,网络的邻居节点之间的信息传输总是不完美的。比如任何通讯网络在单位时间内传输的信息都是有限的并且由于碰撞等因素也会导致数据的丢失,并且信息在传递时,延时等通信也不可避免的存在。另外,网络中的每个节点在信息传输时也会受到在线资源等能量的。所以,对于真实环境中的复杂网络,在信息传输中充分考虑能量、延时、丢包和带宽(量化),寻找网络达到同步与一致性的条件就成为亟需解决的问题。由此出发,本书主要寻求建立一些统一的框架来研究这类问题。本书包括10章,章概述复杂网络和复杂系统动力学模型以及群体行为的定义。第二章和第三章分别探讨带有延时的一般动力学网络的指数同步和一致性问题。第四章和第五章分别针对连续和离散的多智能体网络给出了在一致性研究中处理延时和量化信号的统一方法。第六章、第七章和第十章主要研究了带有能量约束和延时信息的网络一致性问题。第八章和第九章主要研究了带有脉冲信号的耦合网络的同步问题。
目录
Preface
List of Symbols
Chapter 1 Introduction 1
1.1 Background 1
1.2 Research problems 3
1.2.1 Consensus and practical consensus 6
1.2.2 General model description 6
1.3 Mathematical preliminaries 8
1.3.1 Matrices and graphs 8
1.3.2 Signed graphs 9
1.3.3 Quantizer 11
1.3.4 Discontinuous differential equations 12
1.3.5 Some lemmas 13
References 14
Chapter 2 Consensus over Directed Static Networks with Arbitrary Finite Communication Delays 20
2.1 Linear coupling 21
2.1.1 The case of leaderless 21
2.1.2 The case with one well-informed leader 23
2.2 Nonlinear coupling 25
2.3 Hierarchical structure 27
2.4 Numerical examples 28
2.5 Summary 32
References 32
Chapter 3 Practical Consensus of Multi-Agent Networks with Communication Constraints 35
3.1 Practical consensus with quantized data 36
3.1.1 Model description 36
3.1.2 Finite-time practical consensus under quantization 37
3.1.3 Numerical example 41
3.2 Consensus with hybrid communication constraints 42
3.2.1 Model description and preliminaries 42
3.2.2 The existence of the Filippov solution 43
3.2.3 Practical consensus under quantization and time delay 46
3.2.4 Numerical example 59
3.2.5 Discussions 61
3.3 Summary 64
References 65
Chapter 4 Multi-Agent Consensus with Quantization and Communication Delays 67
4.1 Discrete-time case 67
4.1.1 Model description 68
4.1.2 Main results 68
4.1.3 Numerical example 73
4.2 Continuous-time case 74
4.2.1 Model description and preliminaries 74
4.2.2 The existence of the Filippov solution 75
4.2.3 Consensus analysis under quantization and time delays 77
4.2.4 Numerical example 86
4.3 Summary 87
References 87
Chapter 5 Event-Based Network Consensus with Communication Delays 89
5.1 Distributed discrete-time event-triggered consensus with delays 89
5.1.1 Model description 90
5.1.2 Distributed event-triggered approach 91
5.1.3 Numerical example 97
5.2 Distributed continuous-time event-triggered consensus with delays 99
5.2.1 Model description 99
5.2.2 Asynchronously distributed event-triggered approach 100
5.2.3 Synchronously event-triggered control 107
5.2.4 Numerical example 110
5.3 Summary 111
References 112
Chapter 6 Consensus of Networked Multi-Agent Systems with Antagonistic Interactions and Communication Delays 114
6.1 Continuous-time multi-agent consensus 115
6.1.1 Linear coupling 115
6.1.2 Nonlinear coupling 121
6.1.3 Numerical examples 124
6.2 Discrete-time multi-agent consensus 127
6.2.1 Distributed event-based bipartite consensus 127
6.2.2 Self-triggered approach 140
6.2.3 Numerical example 143
6.3 Summary 146
References 146
Chapter 7 Finite-Time and Fixed-Time Bipartite Consensus for Multi-Agent Systems with Antagonistic Interactions 148
7.1 Preliminaries 150
7.2 Finite-time bipartite consensus 151
7.2.1 Finite-time bipartite consensus protocol 151
7.2.2 Pinning bipartite consensus protocol 156
7.2.3 Numerical examples 159
7.3 Fixed-time bipartite consensus 163
7.3.1 General fixed-time bipartite consensus 165
7.3.2 Signed-average fixed-time bipartite consensus 168
7.3.3 Numerical examples 171
7.4 Summary 173
References 174
Chapter 8 Globally Exponential Synchronization and Synchronizability for General Dynamical Networks 178
8.1 Preliminaries 179
8.2 Synchronization analysis 181
8.2.1 Irreducible case 181
8.2.2 Reducible case 189
8.3 Numerical examples 193
8.4 Summary 200
References 200
Chapter 9 Pinning Cluster Synchronization in an Arrayof Coupled Neural Networks under Event-Based Mechanism 203
9.1 Preliminaries and problem formulation 204
9.2 Pinning cluster synchronization under event-triggered mechanism 207
9.3 Pinning cluster synchronization under self-triggered mechanism 214
9.4 Numerical example 217
9.5 Summary 222
References 222
Chapter 10 Multi-Agent Consensus Recovery Approach under Node Failure 225
10.1 Preliminaries 225
10.2 Consensus analysis of general multi-agent networks 227
10.3 Consensus recovery approach 232
10.4 Numerical examples 239
10.5 Summary 244
References 244
Chapter 11 Conclusion and FutureWork 246
11.1 Conclusion 246
11.2 Future work 247
Color Illustrations
节选
Chapter 1 Introduction 1.1 Background With the rapid development of modern technology, the world has entered the age of networks. Typical examples of networks include the World Wide Web, routes of airlines, biological networks, human relationships, and so on [1]. As a special kind of network, complex networked systems consisting of large groups of cooperating agents have made a significant impact on a broad range of applications including cooperative control of autonomous underwater vehicles (AUVs) [2],scheduling of automated highway systems [3],and congestion control in communication networks [4]. The study of complex networks can be traced back to Euler’s celebrated solution of the Konigsberg bridge problem in 1735,which is often regarded as the first true proof in the theory of networks. In the later 1950s, a random-graph model was proposed by Paul Erdos and Alfred Renyi [5], which laid a solid foundation for modern network theory. Watts and Strogatz proposed a model of small-world networks in 1998 [6]; after that, Barabasi and Albert proposed a model of scale-free networks in 1999 based on the preferential attachment [7]. These two works reveal small-world effect and scale-free property of complex networks and the reasons for the above phenomena. Over the past two decades, complex dynamical networks have been widely exploited by researchers in various fields of physics [8],mathematics [9], engineering [10,11],biology [12],and sociology [13]. What makes complex networked systems distinct from other kinds of systems is that they make it possible to deploy a large number of subsystems as a team to cooperatively carry out a prescribed task. Furthermore, the most striking feature that can be observed in complex networked systems is their ability to show collective behavior that cannot be well explained in terms of the individual dynamics of each single node. Two significant kinds of cooperative behaviors are synchronization and consensus [9,14-18],both of which mean that all agents reach an agreement on certain quantities of interest. The formal study of consensus dates back to 1974 [19], where a mathematical model was presented to describe how the group reaches an agreement. Another interesting discovery is the collective behavior of a group of birds exhibited in foraging or flight, which is found by biologists in the observation of birds’ flocking [20]. If attention is paid, one can find that consensus is a universal phenomenon in nature, such as the shoaling behavior of fish [21], the synchronous flashing of fireflies [22], the swarming behavior of insects [20,23, 24], and the herd behavior of land animals [25]. The key feature of consensus is how local communications and cooperations among agents,i.e., consensus protocols (or consensus algorithms), can lead to certain desirable global behavior [26-29]. Various models have been proposed to study the mechanism of multi-agent consensus problem [30-37]. In [38], the consensus problem was considered of a switched multi-agent system composed of continuous-time and discrete-time subsystems. The authors in [39] investigated consensus problems of a class of second-order continuous-time multi-agent systems with time-delay and jointly-connected topologies. Literature [40] focused on the mean square practical leader-following consensus of second-order nonlinear multiagent systems with noises and unmodeled dynamics. Synchronization, as typical collective behavior and basic motion in nature, means that the difference among the states of any two different subsystems goes to zero as time goes to infinity or time goes to a certain fixed value. Synchronization phenomena exist widely and can be found in different forms in nature and man-made systems, such as fireflies, synchronous flashing, attitude alignment, and the synchronized applause of audiences. To reveal the mechanism of synchronization of complex dynamical networks, a vast volume of work has been done over the past few years. Before the appearance of small-world [6] and scale-free [7] network models, Wu and Chua in [41,42] investigated synchronization of an array of linearly coupled systems and gave some effective synchronization criteria. In 1998, Pecora and Carroll [43] proposed the concept of master stability function as synchronization criterion, which revealed that synchronization highly depends on the coupling strategy or the topology of the network. In [14, 44-46], synchronization in small-world and scale-free networks were studied in detail. Over the past few years, different kinds of synchronization have been found and studied, such as complete synchronization [14,41,42,47,48], cluster synchronization [49-52], phase synchronization [53], lag synchronization [54,55] and generalized synchronization [56]. In the literature, most works on the consensus/synchronization of complex networks mainly focus on the analysis of network models with perfect communication, in which it
-
造神:人工智能神话的起源和破除 (精装)
¥32.7¥88.0 -
大数据技术导论(第2版)
¥28.9¥41.0 -
人工智能
¥20.3¥55.0 -
人人都能学AI
¥40.4¥68.0 -
数据结构基础(C语言版)(第2版)
¥41.7¥49.0 -
系统架构设计师教程(第2版)(全国计算机技术与软件专业技术资格(水平)考试指定用
¥102.7¥158.0 -
过程控制技术(第2版高职高专规划教材)
¥27.6¥38.0 -
WPS OFFICE完全自学教程(第2版)
¥97.3¥139.0 -
智能视频目标检测与识别技术
¥43.5¥59.0 -
人工智能基础及应用
¥36.0¥48.0 -
深入浅出软件架构
¥117.2¥186.0 -
工业互联网安全创新技术及应用
¥79.4¥128.0 -
计算机网络基础(微课版)
¥39.0¥55.0 -
零信任架构
¥62.4¥89.0 -
剪映:即梦AI绘画与视频制作从新手到高手
¥66.0¥89.0 -
红蓝攻防 技术与策略(原书第3版)
¥95.9¥139.0 -
Web前端开发基础
¥37.5¥57.0 -
软件设计的哲学(第2版)
¥52.0¥69.8 -
人工智能的底层逻辑
¥58.7¥79.0 -
软件工程理论与案例
¥63.4¥99.0