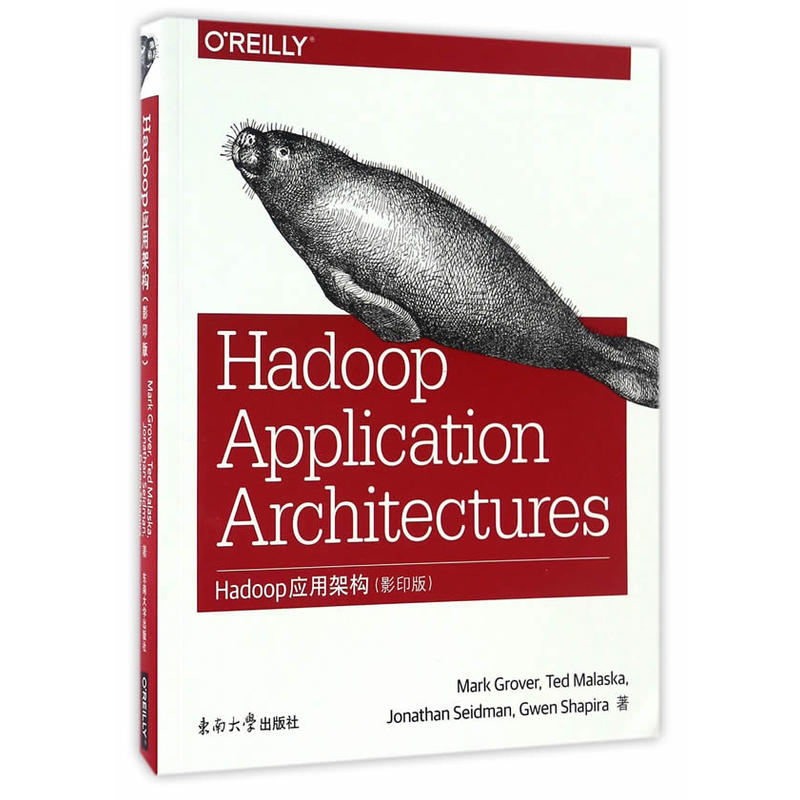
Hadoop应用架构-(影印版)

温馨提示:5折以下图书主要为出版社尾货,大部分为全新(有塑封/无塑封),个别图书品相8-9成新、切口有划线标记、光盘等附件不全详细品相说明>>
- ISBN:9787564170011
- 装帧:暂无
- 册数:暂无
- 重量:暂无
- 开本:32开
- 页数:371
- 出版时间:2017-02-01
- 条形码:9787564170011 ; 978-7-5641-7001-1
本书特色
在使用Apache
Hadoop设计端到端数据管理解决方案时获得专家级指导。当其他很多渠道还停留在解释Hadoop生态系统中该如何使用各种纷繁复杂的组件时,这本专注实践的书已带领你从架构的整体角度思考,它对于你的特别应用场景而言是必不可少的,将所有组件紧密结合在一起,形成完整有针对性的应用程序。
为了增强学习效果,本书第二部分提供了各种详细的架构案例.涵盖部分*常见的Hadoop应用场景。
无论你是在设计一个新的Hadoop应用还是正计划将
Hadoop整合到现有的数据基础架构中,Mark Grover 、Ted Malaska、Jonathan Seidman、Gwen
Shapira编*的《Hadoop应用架构(影印版)(英文版) 》都将在这整个过程中提供技巧性的指导。
使用Hadoop存放数据和建模数据时需要考虑的要素
在系统中导入数据和从系统中导出数据的*佳实践指导 数据处理的框架,包括MapReduce、Spark和 Hive
常用Hadoop处理模式,例如移除重复记录和使用窗口分析 Giraph,GraphX以及其他Hadoop上的大图片处理工具
使用工作流协作和调度工具,例如Apache Oozie 使用Apache Storm、Apache Spark Streaming 和Apache
Flume处理准实时数据流 点击流分析、欺诈防止和数据仓库的架构实例
内容简介
在使用 Apache Hadoop 设计端到端数据管理解决方案时,获得专家级指导。当其它很多渠道还停留在解释 Hadoop 生态系统中该如何使用各种纷纭复杂的组件时,这本专注实践的书已带领您从架构的整体角度思考,这样的角度对于您的特别应用场景而言,是必不可少的。它将所有组件紧密结合在一起,形成完整有针对性的应用程序。为了增强学习效果,本书第二部分提供了各种详细的架构案例,涵盖部分*常见的 Hadoop 应用场景。无论您在设计一个新的 Hadoop 应用,或者正计划将 Hadoop 整合到现有的数据基础架构中,本书都将在整个过程中提供技巧性的导引。
目录
-
有限与无限的游戏:一个哲学家眼中的竞技世界
¥37.4¥68.0 -
全图解零基础word excel ppt 应用教程
¥12.0¥48.0 -
机器学习
¥59.4¥108.0 -
深度学习的数学
¥43.5¥69.0 -
智能硬件项目教程:基于ARDUINO(第2版)
¥37.7¥65.0 -
硅谷之火-人与计算机的未来
¥14.3¥39.8 -
元启发式算法与背包问题研究
¥38.2¥49.0 -
AI虚拟数字人:商业模式+形象创建+视频直播+案例应用
¥62.9¥89.8 -
UNIX环境高级编程(第3版)
¥164.9¥229.0 -
剪映AI
¥52.8¥88.0 -
深度学习高手笔记 卷2:经典应用
¥90.9¥129.8 -
纹样之美:中国传统经典纹样速查手册
¥77.4¥109.0 -
UG NX 12.0数控编程
¥24.8¥45.0 -
MATLAB计算机视觉与深度学习实战(第2版)
¥90.9¥128.0 -
界面交互设计理论研究
¥30.8¥56.0 -
UN NX 12.0多轴数控编程案例教程
¥25.8¥38.0 -
微机组装与系统维护技术教程(第二版)
¥37.8¥43.0 -
明解C语言:实践篇
¥62.9¥89.8 -
Linux服务器架设实战(Linux典藏大系)
¥84.5¥119.0 -
PREMIERE PRO 2023全面精通:视频剪辑+颜色调整+转场特效+字幕制作+案例实战
¥69.3¥99.0