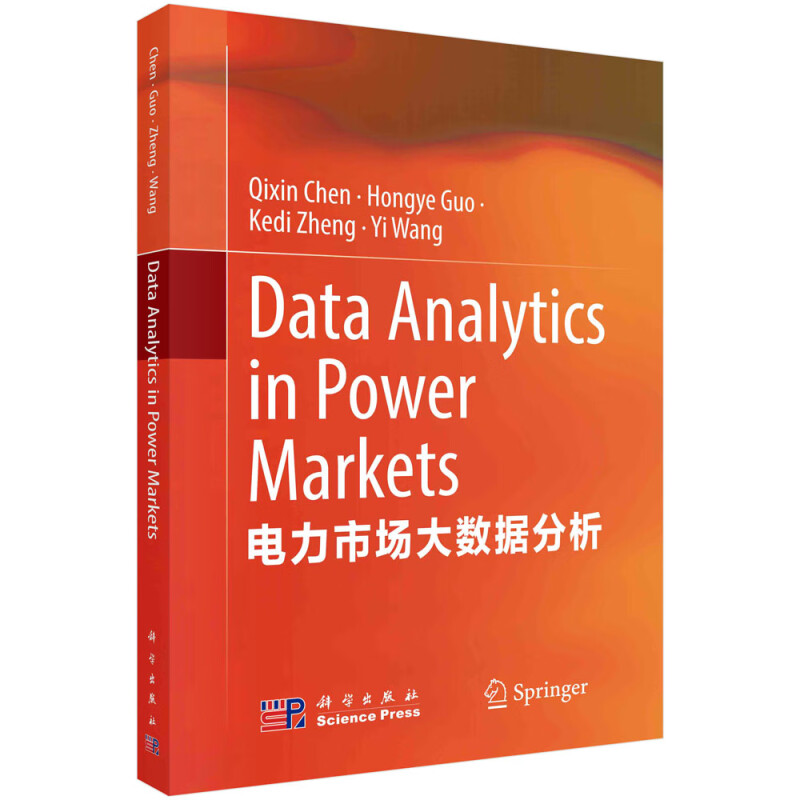
包邮电力市场大数据分析(英文版)

- ISBN:9787030715166
- 装帧:一般胶版纸
- 册数:暂无
- 重量:暂无
- 开本:B5
- 页数:304
- 出版时间:2022-10-01
- 条形码:9787030715166 ; 978-7-03-071516-6
本书特色
Undergraduatestudent,graduatestudent,powersystemengineerandparticipant
内容简介
Thisbookaimstosolvesomekeyproblemsinthedecisionandoptimizationprocedureforpowermarketorganizersandparticipantsindata-drivenapproaches.Itbeginswithanoverviewofthepowermarketdataandanalyzesontheircharacteristicsandimportanceformarketclearance.Thenitdiscussestheessentialproblemofbusloadforecastingfromtheperspectiveofmarketorganizers.Therelatedworksincludingloaduncertaintymodeling,busloadbaddatacorrection,andmonthlyloadforecasting.Thefollowingworkstrytomodelthecomplexmarketbiddingbehaviors.Specificworksincludepatternextraction,aggregatedsupplycurvesforecasting,marketsimulation,andrewardfunctionidentificationinbidding.
目录
1 Introduction to Power Market Data 1
1.1 Overview of Electricity Markets 1
1.2 Organization and Data Disclosure of Electricity Market 4
1.2.1 Transaction Data 5
1.2.2 Price Data 7
1.2.3 Supply and Demand Data 7
1.2.4 System Operation Data 8
1.2.5 Forecast Data 8
1.2.6 Confidential Data 9
1.3 Conclusions 9
References 9
PartⅠ Load Modeling and Forecasting
2 Load Forecasting with Smart Meter Data 13
2.1 Introduction 13
2.2 Framework 14
2.3 Ensemble Learning for Probabilistic Forecasting 16
2.3.1 Quantile Regression Averaging 17
2.3.2 Factor Quantile Regression Averaging 18
2.3.3 LASSO Quantile Regression Averaging 18
2.3.4 Quantile Gradient Boosting Regression Tree 19
2.3.5 Rolling Window-Based Forecasting 20
2.4 Case Study 20
2.4.1 Experimental Setups 2
2.4.2 Evaluation Criteria 21
2.4.3 Experimental Results 22
2.5 Conclusions 24
References 24
3 Load Data Cleaning and Forecasting 27
3.1 Introduction 27
3.2 Characteristics of Load Profiles 29
3.2.1 Low-Rank Property of Load Profiles 29
3.2.2 Bad Data in Load Profiles 30
3.3 Methodology 31
3.3.1 Framework 31
3.3.2 Singular Value Thresholding (SVT) 32
3.3.3 Quantile RF Regression 34
3.3.4 Load Forecasting 35
3.4 Evaluation Criteria 35
3.4.1 Data Cleaning-Based Criteria 35
3.4.2 Load Forecasting-Based Criteria 35
3.5 Case Study 36
3.5.1 Result of Data Cleaning 36
3.5.2 Day Ahead Point Forecast 37
3.5.3 Day Ahead Probabilistic Forecast 38
3.6 Conclusions 40
References 40
4 Monthly Electricity Consumption Forecasting 43
4.1 Introduction 43
4.2 Framework 46
4.2.1 Data Collection and Treatment 46
4.2.2 SVECM Forecasting 47
4.2.3 Self-adaptive Screening 48
4.2.4 Novelty and Characteristics of SAS-SVECM 48
4.3 Data Collection and Treatment 48
4.3.1 Data Collection and Tests 49
4.3.2 Seasonal Adjustments Based on X-12-ARIMA 49
4.4 SVECM Forecasting 49
4.4.1 VECM Forecasting 49
4.4.2 Time Series Extrapolation Forecasting 52
4.5 Self-adaptive Screening 53
4.5.1 Influential EEF Identification 53
4.5.2 Influential EEF Grouping 53
4.5.3 Forecasting Performance Evaluation Considering Different EEF Groups 55
4.6 Case Study 56
4.6.1 Basic Data and Tests 56
4.6.2 Electricity Consumption Forecasting Performance Without SAS 58
4.6.3 EC Forecasting Performance with SAS 61
4.6.4 SAS-SVECM Forecasting Comparisons with Other Forecasting Methods 65
4.7 Conclusions 67
References 67
5 Probabilistic Load Forecasting 71
5.1 Introduction 71
5.2 Data and Model 73
5.2.1 Load Dataset Exploration 73
5.2.2 Linear Regression Model Considering Recency-Effects 73
5.3 Pre-Lasso Based Feature Selection 76
5.4 Sparse Penalized Quantile Regression (Quantile-Lasso) 77
5.4.1 Problem Formulation 77
5.4.2 ADMM Algorithm 78
5.5 Implementation 80
5.6 Case Study 81
5.6.1 Experiment Setups 81
5.6.2 Results 82
5.7 Concluding Remarks 86
References 86
Part Ⅱ Electricity Price Modeling and Forecasting
6 Subspace Characteristics of LMP Data 91
6.1 Introduction 91
6.2 Model and Distribution of LMP 93
6.3 Methodology
6.3.1 Problem Formulation 96
6.3.2 Basic Framework 97
6.3.3 Principal Component Analysis 98
6.3.4 Recursive Basis Search (Bottom-Up) 98
6.3.5 Hyperplane Detection (Top-down) 100
6.3.6 Short Summary 103
6.4 Case Study 103
6.4.1 Case 1: IEEE 30-Bus System 104
6.4.2 Case 2: IEEE 118-Bus System 106
6.4.3 Case 3: Illinois 200-Bus System 106
6.4.4 Case 4: Southwest Power Pool (SPP) 107
6.4.5 Time Consumption 108
6.5 Discussion and Conclusion 110
6.5.1 Discussion on Potential Applications 110
6.5.2 Conclusion 110
References 111
7 Day-Ahead Electricity Price Forecasting 113
7.1 Introduction 113
7.2 Problem Formulation 116
7.2.1 Decomposition of LMP 116
7.2.2 Short-Term Forecast for Each Component 117
7.2.3 Summation and Stacking of Individual Forecasts 118
7.3 Methodology 119
7.3.1 Framework 119
7.3.2 Feature Engineering 121
7.3.3 Regression Model Selection and Parameter Tuning 122
7.3.4 Model Stacking with Robust Regression 123
7.3.5 Metrics 124
7.4 Case Study 124
7.4.1 Model Selection Results 125
7.4.2 Componential Results 126
7.4.3 Stacking Results (Overall Improvements) 128
7.4.4 Error Distribution Analysis 129
7.5 Conclusion 132
References 132
8 Economic Impact of Price Forecasting Error 135
8.1 Introduction 135
8.2 General Bidding Models 137
8.2.1 Deterministic Bidding Model 138
8.2.2 Stochastic Bidding Model 139
8.3 Methodology and Framework 141
8.3.1 Forecasting Error Modeling 141
8.3.2 Multiparametric Linear Programming (MPLP)Theory 141
8.3.3 Error Impact Formulation 142
8.3.4 Overall Framework 144
8.4 Case Study 145
8.4.1 Measurement of STPF Error Level 145
8.4.2 Case 1: LSE with Deman
节选
Chapter 1 Introduction to Power Market Data 1.1 Overview of Electricity Markets Electricity is widely used as a kind of efficient and convenient energy. The trading of electricity not only has typical characteristics of bulk commodity trading but also must meet certain constraints of physical laws. Hogan pointed out that a successful electricity market should be based on the principle of “bid-based,security-constrained economic dispatch (SCED),,[1]. At present, the electricity spot markets in the world (including markets in the U.S., EU, Australia, and other countries, as well as the pilot market in the trial operation stage in China) basically follow this principle. “Bid-based” refers to that market participants need to declare their own cost or utility of generating or purchasing electricity, so as to form the supply and demand curves of the market. The SCED model is usually a linear programming model whose objective function is the maximization of social welfare. The balance of energy, transmission capacity, as well as upper and lower limits of generation are included in the constraints of SCED. The optimal solution determines the cleared capacity of the market participants, and the shadow prices of the constraints are used to calculate the locational marginal prices (LMP), representing the marginal cost of the power system caused by the incremental demand at each node. Figure 1.1 shows the timeline of wholesale electricity markets organized by regional transmission operators (RTOs) and independent system operators (ISOs) in the U.S. and Canada. The deregulation of electricity markets in the U.S. began in the 1990s. At present, there are seven ISO/RTO markets in the U.S., including PJM [3], CAISO [4], NYISO [5], etc., most of which are under the regulation of the Federal Energy Regulatory Commission (FERC) [6], except for the ERCOT [7] in Texas. Take PJM, the earliest deregulated market in the U.S.,as an example [8]. PJM is located in the northeast of the U.S., and its main management area covers Pennsylvania, New Jersey, and Maryland. In 1997,PJM opened its first bid-based energy market. Later that year, the FERC approved PJM as the nation’s first fully functioning ISO. Then, from 1998 to 2001,PJM completed the implementation of LMP mechanism, financial transmission right (FTR), day-ahead market, and ancillary services market of load frequency regulation. PJM became the nation’s first fully functioning RTO in 2002. After 2002,PJM focuses on the integration of other small regional transmission networks and expansion of the wholesale market scale. Currently, the wholesale markets in the U.S. adopt a two-settlement (i.e., day-ahead and real-time settlement) system and use the LMP calculated based on SCED models for payment. The goal of electricity market deregulation in EU is to establish an integrated electricity market for the whole union. As EU has many member countries, its implementation of cross-border market coupling is divided into several steps. In the first step, national markets were created, starting from the partial market opening in 1999. As the next step, interconnectors were forced to open to market-based capacity allocation from 2003. Then, market coupling was established and expanded after 2005 [10]. By 2019, the EU power system had covered 36 countries and 43 transmission system operators (TSO), and the European power exchange center EPEX SPOT [11] is responsible for cross-border transactions as well as day-ahead and intra-day coupling markets. The EU power market has completed unified day-ahead operation of 25 countries and intra-day operation of 14 countries. Compared with the U.S. electricity markets, a significant difference of the EU market is the adoption of zonal price instead of the nodal price scheme, which usually requires sufficient transmission capacity in each zone to avoid congestion and ensure a uniform zonal price. If congestion occurs, the local TSO needs to redispatch the units in the zone and make certain compensation. Figure 1.2 shows the main timeline of electricity market coupling in EU. The recent deregulation of China’s power sector started with the release of the ninth document entitled Several Opinions of the CPC Central Committee and the State Council on Further Deepening the Reform of the Electric Power System in March 2015. At present, the construction and operation of medium and long-term markets have been completed in all provinces of China. The construction of spot markets has almost been finished in eight pilot provinces and will be expanded to other provinces in the future. Currently, the spot markets have entered the trial operation stage in these pilot provinces. The map in Fig. 1.3 shows the timeline for electricity spot market evolution in China. Although there are differences in the detailed rules of these pilot spot markets, the principle in their organization, transaction and settlement is similar. The data analytic m
-
污水处理工程工艺设计从入门到精通
¥70.3¥89.0 -
发电厂电气部分
¥34.6¥58.0 -
植物进化的故事
¥19.9¥59.0 -
赶往火星:红色星球定居计划
¥44.5¥58.0 -
果脯蜜饯加工技术
¥4.5¥12.0 -
数控车工
¥5.9¥11.5 -
数据驱动的剩余寿命预测与维护决策技术
¥63.4¥79.0 -
手术机器人导航与控制
¥127.4¥169.8 -
射频干扰袖珍手册
¥18.4¥29.0 -
汽车车身构造与修复
¥30.7¥45.0 -
群目标分辨雷达初速测量技术
¥42.4¥69.0 -
秸秆挤压膨化技术及膨化腔流道仿真研究
¥40.6¥55.0 -
NVH前沿科技与工程应用
¥109.7¥159.0 -
电力系统分析
¥23.8¥38.0 -
继电保护原理
¥30.4¥49.0 -
不确定条件下装备剩余寿命预测方法及应用
¥60.4¥99.0 -
船舶分段装配
¥58.6¥80.0 -
基于深度学习的复杂退化系统剩余寿命智能预测技术
¥54.4¥89.0 -
火星探测器轨道动力学与控制
¥59.8¥98.0 -
美军联合作战弹药保障
¥35.8¥58.0